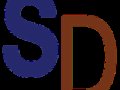
Ionic liquid-based reservoir computing: The key to efficient and flexible edge computing
https://www.sciencedaily.com/releases/2022/04/220428085817.htm
Artificial Intelligence (AI) is fast becoming ubiquitous in the modern society and will feature a broader implementation in the coming years. In applications involving sensors and internet-of-things devices, the norm is often edge AI, a technology in which the computing and analyses are performed close to the user (where the data is collected) and not far away on a centralized server. This is because edge AI has low power requirements as well as high-speed data processing capabilities, traits that are particularly desirable in processing time-series data in real time.
In this regard, physical reservoir computing (PRC), which relies on the transient dynamics of physical systems, can greatly simplify the computing paradigm of edge AI. This is because PRC can be used to store and process analog signals into those edge AI can efficiently work with and analyze. However, the dynamics of solid PRC systems are characterized by specific timescales that are not easily tunable and are usually too fast for most physical signals. This mismatch in timescales and their low controllability make PRC largely unsuitable for real-time processing of signals in living environments.