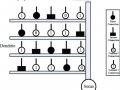
Training with states of matter search algorithm enables neuron model pruning
https://phys.org/news/2018-11-states-algorithm-enables-neuron-pruning.html
However, the well-known backpropagation (BP) algorithm that was used to train the ALMN actually restricted the neuron model's computational capacity. "The BP algorithm was sensitive to initial values and could easily be trapped into local minima," says corresponding author Yuki Todo of Kanazawa University's Faculty of Electrical and Computer Engineering. "We therefore evaluated the capabilities of several heuristic optimization methods for training of the ALMN."
After a series of experiments, the states of matter search (SMS) algorithm was selected as the most appropriate training method for the ALMN. Six benchmark classification problems were then used to evaluate the ALNM's optimization performance when it was trained using the SMS as a learning algorithm, and the results showed that SMS provided superior training performance when compared with BP and the other heuristic algorithms in terms of both accuracy and convergence speed.